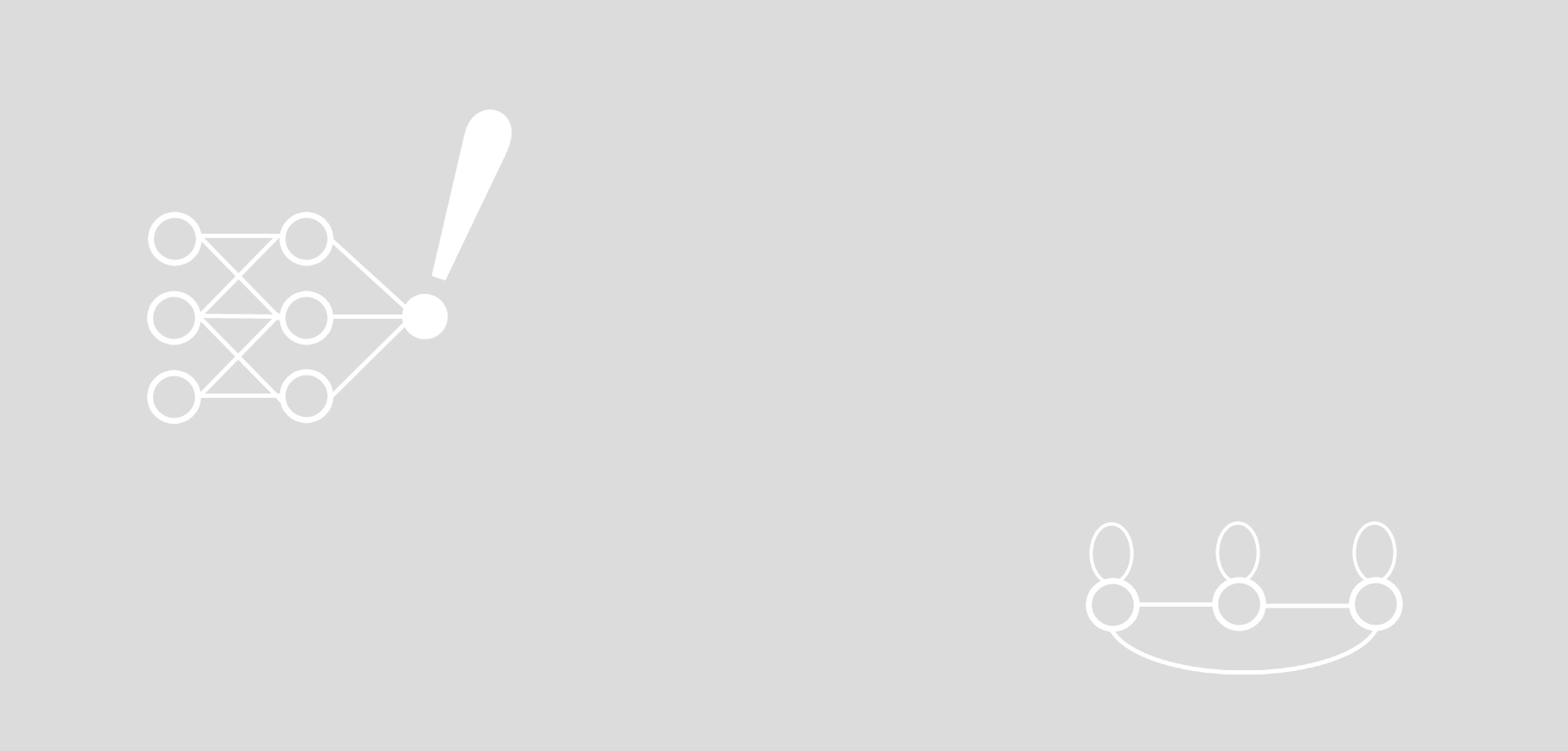
Research
Audio Source Separation
Human can attend individual sounds in their mixture. Extracting the sound of interest is one of the key feature for machine auditory. To achieve this goal, I have been working on sound source separation in various domains, such as music, speech, and universal sounds.
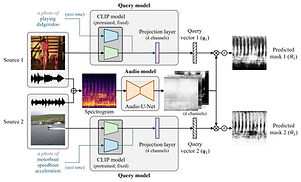
Related papers
-
H. Dong, N. Takahashi*, Y. Mitsufuji, J. McAuley, T. Berg-Kirkpatrick, “CLIPSep: Learning Text-queried Sound Separation with Noisy Unlabeled Videos,” ICLR 2023, *corresponding author [OpenReview][arXiv][demo][code]
-
N. Takahashi, Y. Mitsufuji, “Amicable examples for informed source separation”, ICASSP 2022
-
N. Takahashi, Y. Mitsufuji, “Densely Connected Multi-Dilated Convolutional Networks for Dense Prediction Tasks,” CVPR 2021 [CVF][IEEE][arXiv][code]
-
N. Takahashi, et al., “Improving Voice Separation by Incorporating End-to-end Speech Recognition”, ICASSP 2020
-
N. Takahashi, P. Sudarsanam, N.Goswami, Y. Mitsufuji, “Recursive speech separation for unknown number of speakers”, Interspeech 2019
-
N. Takahashi, P.Agrawal, N.Goswami, Y. Mitsufuji, “PhaseNet: Discretized Phase Modeling with Deep Neural Networks for Audio Source Separation”, Interspeech 2018
-
N. Takahashi, N.Goswami, Y. Mitsufuji, “MMDenseLSTM: an Efficient Combination of Convolutional and Recurrent Neural Networks for Audio Source Separation”, IWAENC 2018
-
N. Takahashi, Y. Mitsufuji, “Multi-scale Multi-band DenseNets for Audio Source Separation”, WASPAA 2017
-
S. Uhlich, M. Porcu, F. Giron, M. Enenkl, T. Kemp, N. Takahashi and Y. Mitsufuji, Improving Music Source Separation Based On Deep Networks Through Data Augmentation And Augmentation And Network Blending, ICASSP 2017
Acoustic Event Recognition

Beside vision, sounds provide complemental information for understanding environments. Recognizing and localizing objects from sounds are essential ability for many animals to live in the real world. Towards machine auditory, I have been working on sound event recognition and localization.
Related papers
-
K. Shimada, Y. Koyama, S. Takahashi, N.Takahashi, E. Tsunoo, Y. Mitsufuji, "Multi-ACCDOA: Localizing and Detecting Overlapping Sounds from the Same Class with Auxiliary Duplicating Permutation Invariant Training", ICASSP 2022 [arXiv]
-
K. Shimada, Y. Koyama, N. Takahashi, S. Takahashi, Y. Mitsufuji, “ACCDOA: Activity-Coupled Cartesian Direction of Arrival Representation for Sound Event Localization and Detection”, ICASSP 2021, [arXiv]
-
N. Takahashi, Michael Gygli, Luc Van Gool, "AENet: Learning deep audio features for video analysis", IEEE Transactions on Multimedia, Vol.20 Issue 3, 2017 [IEEE][arXiv]
-
N. Takahashi, Michael Gygli, Beat Pfister, Luc Van Gool, "Deep Convolutional Neural Networks and Data Augmentation for Acoustic Event Recognition" Interspeech 2016 [arXiv]
Voice Conversion

Voice conversion aim at converting characteristics of source voice while maintaining its content. I have been working on singing on voice conversion that works robustly on vocals separated from accompaniment music. [demo]
Video Analysis
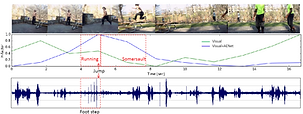
We improve video analysis such as action recognition and video highlight detection by using audio-visual features.
Related papers
-
N. Takahashi, Michael Gygli, Luc Van Gool, "AENet: Learning deep audio features for video analysis", IEEE Transactions on Multimedia, Vol.20 Issue 3, 2017 [IEEE][arXiv][Invited talk]
Automatic Speech Recognition

Automatic speech recognition for low-resource domain remains open question.
Related papers
-
S. Basak, S. Agarwal, S. Ganapathy, N. Takahashi, “End-to-end lyrics Recognition with Voice to Singing Style Transfer” , ICASSP 2021
-
N. Takahashi, T. Naghibi, B. Pfister, "Automatic Pronunciation Generation by Utilizing a Semi-supervised Deep Neural Networks", Interspeech 2016
Semantic Segmentation
Semantic segmentation aims at classifying every pixels of a image into object categories. Semantic segmentation is a dense prediction task and requires efficient modeling in both coarse and fine-grained scales. I have proposed a novel architecture called D3Net, which combines multi-resolution information in all layers while avoiding aliasing problem.
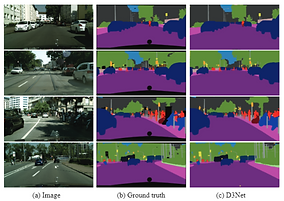